AI Farming Technology: Revolutionizing Agriculture
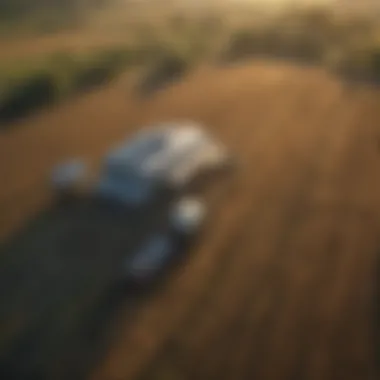
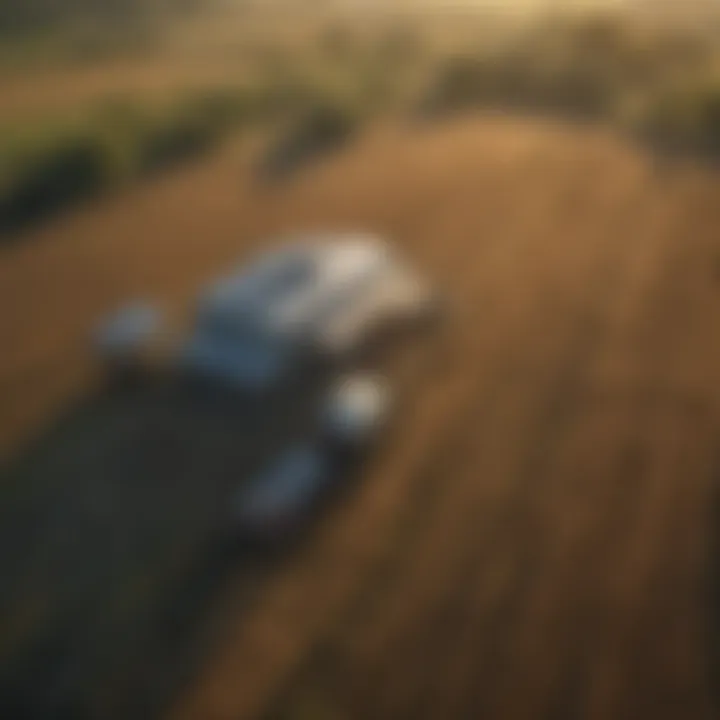
Intro
The role of artificial intelligence in agriculture is on the rise, driven by the need for increased efficiency and sustainability. In a world facing climate change, dwindling resources, and a growing population, AI farming technology provides innovative solutions that directly address these challenges. This exploration unveils how AI revolutionizes traditional farming practices and introduces a new paradigm in agriculture. Understanding the concepts and terminology around AI farming is essential for stakeholders seeking to leverage these advancements.
Key Concepts and Terminology
Basic Definitions
Artificial intelligence in farming involves the integration of algorithms and data analysis to enhance decision-making and efficiency in agricultural practices. Key terms in this domain include:
- Precision Agriculture: This refers to a farming management concept that uses technology to monitor and manage variability in crops.
- Automated Labor: This involves the use of robotics and machinery to perform tasks traditionally done by human labor.
- Data Analytics: The process of examining data sets to uncover patterns or insights that can inform farming practices.
Historical Context
Historically, farming has relied heavily on manual labor and traditional techniques. The agricultural revolution marked a significant shift, introducing mechanization and synthetic fertilizers. As technology evolved, the introduction of information technology helped farmers manage resources more effectively. The recent surge in AI represents the next major leap in this evolution, enhancing capabilities and paving the way for modern, data-driven agriculture.
Recent Innovations and Trends
Technological Advancements
Within the last few decades, several technological advancements have emerged in AI farming:
- Drones: These devices are now commonly used for aerial mapping, crop monitoring, and pest control.
- Sensors: Soil and environmental sensors provide real-time data on moisture levels, nutrient concentrations, and weather patterns.
- Predictive Analytics: Algorithms analyze historical data to forecast crop yields and optimize planting schedules.
Sustainable Practices
Implementing AI in agriculture not only boosts productivity but also promotes sustainable practices:
- Resource Optimization: AI helps farmers use water, fertilizers, and pesticides more efficiently, minimizing waste and environmental impact.
- Crop Rotation Planning: Data can guide rotation practices that preserve soil health and reduce pests.
Practical Applications and Techniques
Step-by-step Guides
To implement AI in farming, farmers can follow these general steps:
- Assess Needs: Identify the specific challenges or areas where AI could offer solutions.
- Select Technology: Choose suitable tools like drones, soil sensors, or AI-powered software.
- Training and Implementation: Ensure staff is trained to use the selected technology effectively.
Case Studies
Analyzing successful applications of AI farming can offer insights into its potential:
- Case Study 1: John Deere's use of AI for precision planting has significantly improved crop yields by optimizing planting density.
- Case Study 2: The approach taken by Climate Corp shows the integration of AI data analytics to predict weather patterns, allowing farmers to plan better.
"The adoption of AI technologies in agriculture is not just about increasing yields; it is about creating a sustainable ecosystem and a future for farming."
In sum, the integration of AI farming technology is poised to reshape agriculture significantly. Its ability to optimize processes and minimize the ecological footprint offers a valid pathway towards sustainable agricultural practices. Understanding these concepts will enable farmers and enthusiasts to navigate this evolving landscape effectively.
As we advance, it remains critical to stay informed about ongoing developments and how they can be applied to improve efficiencies and meet the demands of modern agriculture.
Foreword to AI in Agriculture
In recent years, the agricultural sector has witnessed significant transformations driven by advancements in technology. Artificial Intelligence, often referred to simply as AI, is now at the forefront of this evolution. This section aims to outline the significance of AI farming technology and explore how it plays a crucial role in enhancing agricultural practices. Understanding the core principles of AI in agriculture, its implementation, and the benefits it brings requires careful examination.
AI farming technology encapsulates various tools and methodologies that leverage machine learning, data analytics, and automation to optimize farming operations. This technology offers the potential to increase productivity, manage resources efficiently, and minimize environmental impacts. However, the adoption of such technologies is not without its challenges and considerations. Farmers and stakeholders must be equipped with the knowledge to navigate these complexities.
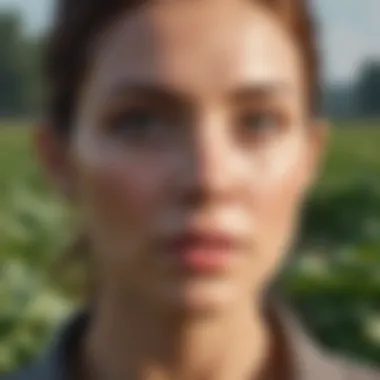
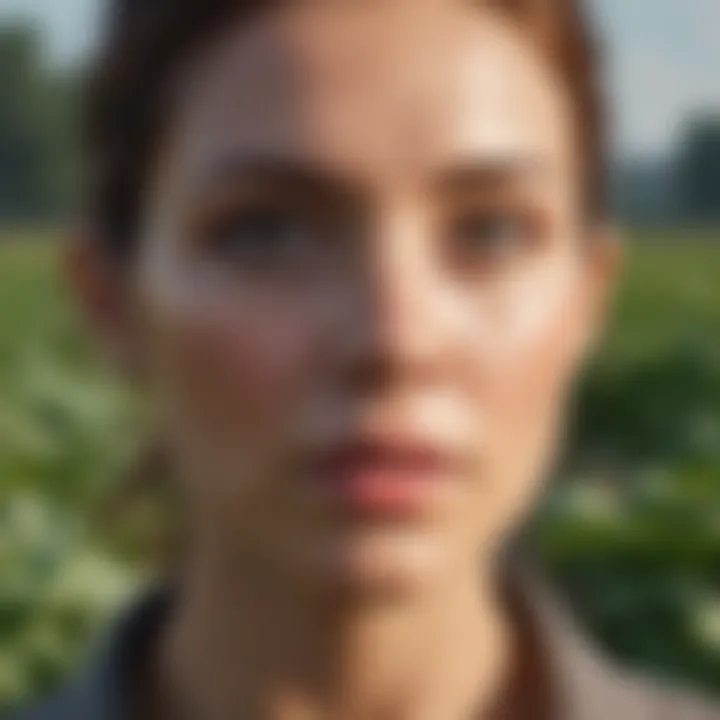
Defining AI Farming Technology
AI farming technology refers to the integration of artificial intelligence principles into agricultural processes. This can include a wide array of applications, from precision agriculture and automated systems to decision-making tools that utilize data analysis. The core component of AI in agriculture is its ability to process vast amounts of information and produce actionable insights. By harnessing machine learning algorithms, farmers can predict crop yields, assess soil health, and identify pest infestations more accurately than ever before.
Furthermore, AI can facilitate real-time monitoring of agricultural conditions, allowing for immediate interventions when necessary. This adaptability is vital for addressing the dynamic nature of farming environments. The definitions can vary based on context and usage, yet the central theme remains consistent: AI enhances the cultivation process, making it more efficient and sustainable.
Historical Context of Agriculture and Technology
To fully appreciate AI farming technology, it is essential to consider its historical context. Agriculture has always been an area of innovation. The introduction of tools like the plow, later the tractor, set the stage for increased productivity and scale. Over centuries, as technology developed, so did the methods of farming.
The advent of the computer and later the Internet further revolutionized agriculture. Data became more accessible, enabling farmers to make data-driven decisions. In the late 20th century, concepts such as precision agriculture began to emerge, incorporating satellite imagery and GPS technology.
As we entered the 21st century, the exponential growth of AI and machine learning provided fresh opportunities. This evolution in technology signifies not just an upgrade in tools, but a paradigm shift in how agricultural challenges are approached. Integrating AI helps farmers not only cope with contemporary issues but also prepares them for the future unpredictabilities of climate change, population growth, and resource constraints.
This historical background sets the stage for the ongoing transformations in agriculture due to AI-driven technologies, revealing both the opportunities available and the responsibilities that come with them.
Components of AI Farming Technology
The segment of AI farming technology encompasses several critical components that work in concert to revolutionize agricultural practices. Understanding these elements is essential for anyone interested in the intersection of technology and agriculture. Each component contributes to enhanced efficiency, improved crop yield, and sustainable farming practices. This section aims to break down these components, illustrating their significance and the current developments in each.
Data Collection and Analysis
Data collection is fundamental in modern agriculture. Sensors, drones, and satellite imaging gather vast amounts of information about soil health, moisture levels, and crop development. This data is invaluable for making informed decisions. Analyzing this data allows farmers to pinpoint trends and anomalies that affect yields. Methods such as remote sensing and geospatial analysis enable farmers to understand their fields' conditions in real-time.
- Sensors can measure soil temperature and pH levels.
- Drones can capture high-resolution images for crop health assessment.
- Satellite data provides insights into weather patterns that influence planting and harvesting schedules.
Such analytical capabilities are changing how farmers approach cultivation strategies. It helps to optimize planting times, watering schedules, and fertilizer application. Accurate data analysis leads to improved productivity and resource management, which is crucial for sustainable agriculture.
Machine Learning Algorithms
Machine learning algorithms play a pivotal role in AI farming technology. They enhance decision-making by learning from historical data and predicting outcomes based on various inputs. These algorithms can analyze data trends over time and suggest specific actions to improve farm operations. For example, they can predict pest infestations, disease outbreaks, or optimal harvest times based on previous years' data.
Benefits of machine learning in agriculture include:
- Yield prediction, helping farmers plan effectively.
- Pest detection, minimizing crop loss through timely interventions.
- Soil health analysis, enabling better fertilization strategies.
The algorithms continuously evolve with more data, improving their accuracy and reliability over time. Implementing these algorithms requires a degree of technical literacy, which may pose a challenge for some farmers, but the evident benefits often outweigh these challenges.
Robotics and Automation
Robotics and automation are at the forefront of AI farming technology. Automated machinery, such as autonomous tractors and robotic harvesters, are becoming more common in the agriculture sector. These machines perform tasks with minimal human intervention, making farming more efficient and cost-effective.
Automation can lead to:
- Reduced labor costs, allowing farmers to allocate resources elsewhere.
- Increased precision, as machines can perform tasks more consistently than humans.
- Enhanced safety, since robots can take on hazardous tasks in fields like pesticide application.
However, the shift toward automation raises questions about employment in agriculture. Farmers must navigate this transition carefully, balancing efficiency improvements with the need for human oversight and labor.
"Adopting AI farming technology will redefine our relationship with the land, leading to greater efficiency and productivity."
Applications of AI in Farming
AI technology has become a crucial element in modern agriculture. The integration of AI enhances the ability of farmers to make data-driven decisions. This section outlines how AI applications are influencing farming practices. By focusing on specific elements like precision agriculture, crop monitoring, and predictive analytics, we create a comprehensive understanding of their impact.
Precision Agriculture
Benefits of Precision Farming
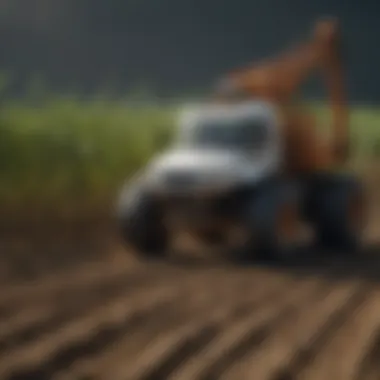
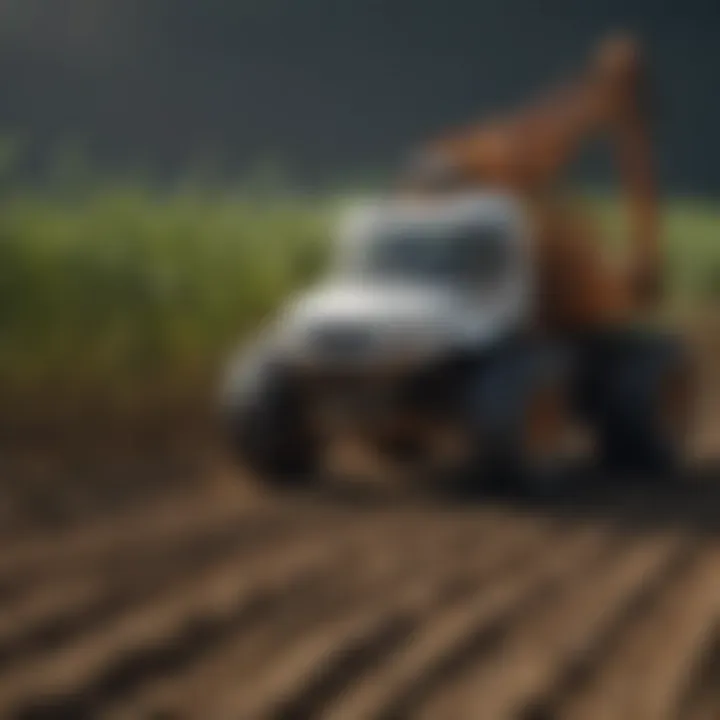
Precision farming optimizes field variability in crops. By utilizing GPS and IoT technology, farmers can gather detailed data. This is significant as it enables tailored agricultural practices. Through real-time monitoring, adjustments can be made to irrigation and fertilization.
One key characteristic of precision farming is its ability to increase efficiency. Farmers can reduce waste of resources like water and fertilizer. This not only saves costs but also benefits the environment. Special features, such as drones and sensors, help in assessing crop health. However, high initial investment can be a barrier.
Case Studies in Precision Agriculture
Examining real-life applications shows the effectiveness of precision agriculture. For instance, John Deere provides examples of farmers using smart machinery. These cases highlight the remarkable benefits in yield improvement.
The unique feature of case studies is their practical insight into implementation. They demonstrate how technology translates to real-world benefits. Learning from these instances can guide new adopters. However, challenges like data management remain a topic of concern.
Crop Monitoring and Management
AI empowers farmers in overseeing their crops efficiently. Using satellite imagery and local sensors, monitoring assists in identifying issues early. This proactive approach helps to mitigate risks to yield.
With machine learning, patterns can be recognized, leading to better management practices. Specific algorithms help predict crop diseases, guiding timely interventions. Active monitoring contributes to healthier plants and better overall farm productivity.
Predictive Analytics for Yield Forecasting
Predictive analytics plays a pivotal role in shaping agricultural strategies. By analyzing historical data, algorithms can offer predictions on crop yields. Farmers can use this information to plan their resources more effectively.
The significance lies in its impact on food supply chains. Accurate forecasts help in aligning production with market demand. This can lead to enhanced profitability and reduced waste.
"The predictive capabilities of AI not only influence yield outcomes but also contribute to sustainability in farming practices."
In summary, background studies illustrate the potential of leveraging AI in agriculture. The applications not only streamline operations but also encourage a more sustainable approach to farming.
AI-Driven Decision-Making in Agriculture
AI-driven decision-making is reshaping the landscape of agriculture. It empowers farmers to make informed choices based on real-time data analysis and predictive analytics. By leveraging artificial intelligence, farmers can optimize operations, increase crop yields, and minimize wastage. This integration is essential for modern agriculture, especially as global food demand continues to rise alongside environmental concerns.
The role of AI in decision-making encompasses various aspects, such as enhancing strategies for pest control, irrigation management, and crop rotation. These methods promote efficiency in daily operations while allowing farmers to respond more effectively to changing conditions.
Optimizing Resource Allocation
One major benefit of AI in agriculture is optimized resource allocation. Traditional farming methods often lead to overuse or underuse of critical resources like water, fertilizers, and pesticides. AI tools analyze historical data and real-time environmental conditions to suggest precise application rates of these inputs.
- Data-Driven Decisions: Farmers can monitor soil moisture levels, weather patterns, and crop health indicators. By accurately interpreting this data, AI leaders can provide tailored recommendations for irrigation schedules or fertilizer types.
- Cost Savings: Improved allocation reduces unnecessary expenditure on inputs. For example, precise water usage not only saves costs but also ensures sustainable practices.
- Yield Improvement: With optimal resource utilization, farmers can enhance productivity, resulting in higher yields.
Reducing Environmental Impact
Reducing the environmental impact of agricultural practices is another crucial advantage of AI-driven decision-making. Traditional methods often harm ecosystems through pesticide overuse and soil degradation.
AI assists in reducing this impact in several ways:
- Targeted Applications: By using AI to identify pest outbreaks, farmers can apply pesticides only where necessary. This practice minimizes chemical runoff and protects beneficial insects.
- Sustainable Practices: The technology promotes crop rotation and diversified planting strategies. These methods improve soil health and biodiversity, which are vital for long-term agricultural sustainability.
- Carbon Footprint: AI-driven optimizations can lead to a decrease in fuel consumption, reducing greenhouse gas emissions during farming operations.
Challenges and Limitations of AI in Agriculture
AI technology holds great promise for modern agriculture, but recognizing its challenges is crucial for informed implementation. These obstacles can affect the efficacy of AI solutions, potentially undermining the advantages they offer. Understanding these difficulties ensures that stakeholders can address them proactively. This section delves into two primary challenges: economic barriers and technical limitations, including data privacy concerns.
Economic Barriers to Adoption
One significant hurdle in adopting AI farming technology is the economic aspects. Many farms, especially small and family-run operations, face financial constraints. The initial investment for AI-based solutions can be substantial. This includes the cost of equipment, software, and training personnel.
In many regions, farmers may not have access to financing options that cater specifically to technology upgrades. Hence, they might be unwilling or unable to invest in AI farming technology, preferring to stick to traditional methods that require lower capital.
Moreover, the return on investment is not always immediate. Achieving noticeable efficiency improvements or crop yields may take time. Farmers need to weigh the potential long-term benefits against immediate costs. The uncertainty of market conditions also complicates this decision, adding to the financial risks involved. In this climate, economic barriers deter some from embracing AI in agriculture.
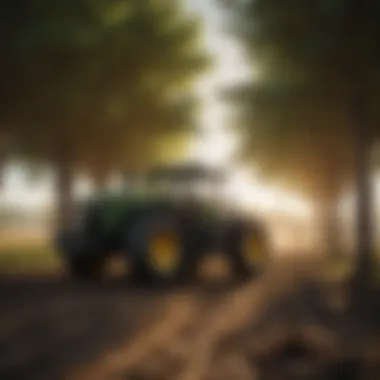
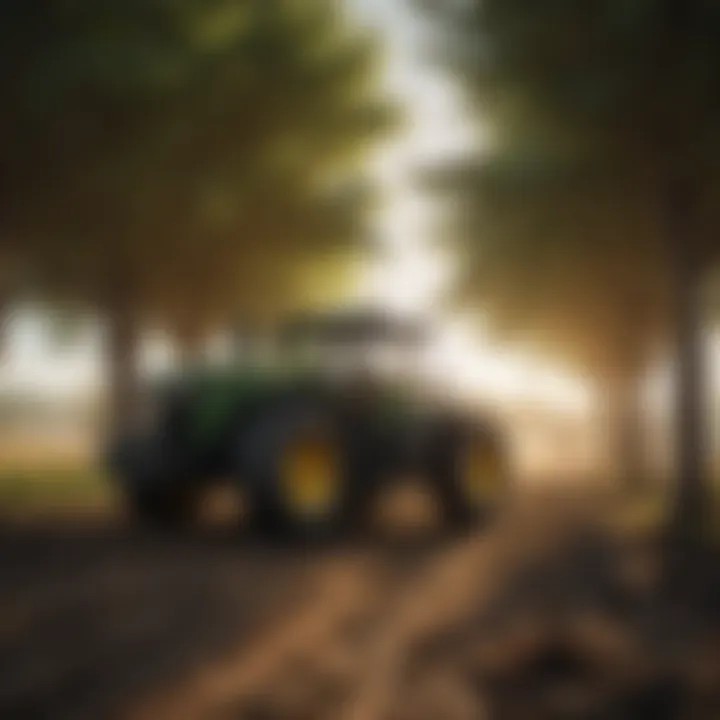
Technical Limitations and Data Privacy
Technical limitations represent another challenge facing AI in agriculture. AI systems require accurate and high-quality data to function effectively. However, many farms lack the necessary infrastructure for comprehensive data collection and analysis. Limited access to sensors, cloud computing, and internet connectivity can inhibit the implementation of AI solutions. Moreover, when farms employ AI, there is reliance on machine learning algorithms to analyze data. If these algorithms are not well-trained, the results may be misleading or inefficient.
Data privacy remains a critical concern in implementing AI farming technology. The collection of vast amounts of data can lead to potential misuse or unauthorized access. Farmers need to be cautious about sharing data with AI systems. There are fears that sensitive information could be exploited, leading to ethical and legal challenges. Lawmakers and technology providers must address these concerns to foster trust in AI tools among farmers.
Future Trends in AI Farming Technology
The landscape of agriculture is on the brink of upheaval due to advancements in AI farming technology. Foresight into future trends shapes the strategies for farmers and stakeholders alike, guiding them towards a more efficient and sustainable approach. Acknowledging these trends is crucial for understanding how technological innovations will not only affect productivity but also redefine the agricultural ecosystem.
Integration with IoT Devices
IoT, or Internet of Things, plays an essential role in bolstering AI capabilities in agriculture. The integration of IoT devices allows for seamless data collection across different farming operations. These devices range from soil moisture sensors to crop health monitoring cameras. The data collected can be analyzed in real-time, enabling immediate response to environmental changes. Moreover, IoT systems facilitate better resource management by providing insights into water usage, fertilizer needs, and pest control measures.
For example, precision irrigation systems that utilize IoT can determine when and how much water to apply, which conserves water and optimizes crop health. In turn, this leads to increased yields without over-exerting resources.
- Key benefits of IoT in agriculture:
- Improved resource allocation
- Enhanced crop monitoring
- Data-driven decision making
The Rise of Autonomous Farming Equipment
The shift towards autonomous farming equipment reflects a significant trend in the agricultural domain. Manufacturers are developing tractors, harvesters, and drones that operate without direct human intervention. These machines are equipped with sensors and AI that enable them to perform tasks such as planting, fertilization, and harvesting with precision.
Embracing automation not only reduces labor costs but also minimizes human error, resulting in higher efficiency. Furthermore, such equipment can operate in a variety of weather conditions and during hours when human workers may not be available, thus extending operational capacities.
With these advancements, farmers can also gather data that helps refine their practices over time. Insights from autonomous equipment can feed back into broader farm management systems, leading to more informed and strategic agricultural practices.
"The future of farming lies in automation and connectivity, with technologies converging to reshape how we approach agriculture."
As these trends continue to evolve, the intersection of AI with IoT and automation heralds a new era of farming. It emphasizes efficiency, sustainability, and data-driven practices that could mitigate some of the pressing challenges facing the agricultural sector today.
Ethical Considerations in AI Agriculture
As artificial intelligence becomes more integrated into agricultural practices, ethical considerations are more relevant than ever. This section seeks to explore the implications of AI in agriculture, focusing on aspects like data ownership and the impact on employment. Understanding these ethical dimensions is vital for ensuring that the adoption of AI technologies is aligned with societal values and agricultural principles.
Data Ownership and Sharing
Data is a critical asset in AI farming technology. It drives algorithms, informs decisions, and enhances productivity. However, the question of who owns this data remains contentious. Farmers often generate valuable data through sensors, GPS technology, and other means. But when they use proprietary software from companies like John Deere or AG Leader Technology, the ownership of this data can become murky.
- Farmers' Rights: Farmers need clarity on their rights to the data they produce. As AI systems collect vast amounts of information, the risks of unauthorized use and data exploitation increase. Understanding ownership rights and ensuring that farmers retain control over their information are essential to fostering a fair and equitable system.
- Sharing and Collaboration: When data is shared, it can enhance community practices and lead to more significant advances in AI agricultural technologies. However, concerns about privacy and misuse might inhibit willing participation. Establishing guidelines for data sharing that prioritize transparency and security can be beneficial.
"The value of data in agriculture is immense, but so are the ethical considerations around its ownership and use."
Impact on Employment in Agriculture
The integration of AI technologies in farming presents both opportunities and challenges for employment in the sector. As automation becomes widespread, the need for traditional labor may decrease, leading to fears about job losses. However, the conversation should account for potential job transformation rather than solely displacement.
- Job Displacement vs. Transformation: While certain roles may diminish, AI also creates new roles that require different skills. For example, positions in data analysis, machine maintenance, and software development will rise as traditional farming tasks become automated. Thus, it is crucial to focus on skill development and training programs to prepare workers for the future of agriculture.
- Quality of Employment: The introduction of AI technologies can improve working conditions. Automated systems can reduce the physical burden of labor, potentially leading to safer and more efficient work environments. In this light, the focus should be on how AI can create higher quality jobs, rather than just the number of jobs available.
Finale
The conclusion of this article serves as a pivotal reflection on the transformative potential of AI in agriculture. It is essential to recognize that the integration of artificial intelligence technologies into farming practices is not merely a trend but a critical evolution in how we approach food production. This evolution encompasses numerous benefits that demand attention.
One of the significant elements highlighted throughout this article is the increase in efficiency AI brings to the agricultural sector. By leveraging advanced data collection and machine learning algorithms, farmers can monitor crop health, optimize resource allocation, and ultimately increase yield. These efficiencies are crucial not only for meeting the growing global food demand but also for ensuring profitability in an increasingly competitive market.
Moreover, there is an intrinsic link between AI farming technology and sustainability. Innovations such as precision agriculture allow for improved environmental stewardship. Farmers can minimize waste, reduce chemical use, and optimize water consumption, leading to a lower ecological footprint. These considerations are vital not only for todayβs farmers but for future generations as well.
However, the adoption of AI is not without its challenges, such as economic barriers and ethical concerns. Farmers must navigate these complex issues as they move towards a tech-oriented future. It becomes imperative for stakeholders to address these challenges collaboratively to create an environment conducive for the widespread adoption of these technologies.
Thus, the path forward for AI in agriculture necessitates balanced approaches that consider both technological advancement and ethical implications. Investing in education and training will empower farmers to effectively utilize AI tools, ensuring a more resilient agricultural framework. The amalgamation of technology and tradition presents a unique opportunity to innovate without losing sight of the needs of the land and the community.
"The true success of AI in agriculture hinges on our capacity to harmonize technology with our inherent understanding of ecological sustainability and community dynamics."